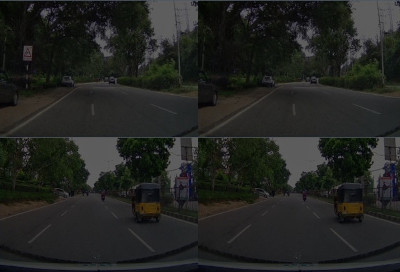
The international Autonomous Vehicle (AV) market is estimated to reach around two thousand billion by 2030. Millions of lives are lost yearly in road accidents, and traffic violations cause a significant percentage of AV accidents. The traffic signs are generally installed at the side of the road to control traffic flow or pass information about the road environment to Vulnerable Road Users (VRUs). Often, the information is also available in the form of cues present in the context around the traffic signs in the cues away from it, which we refer to as contextual cues.